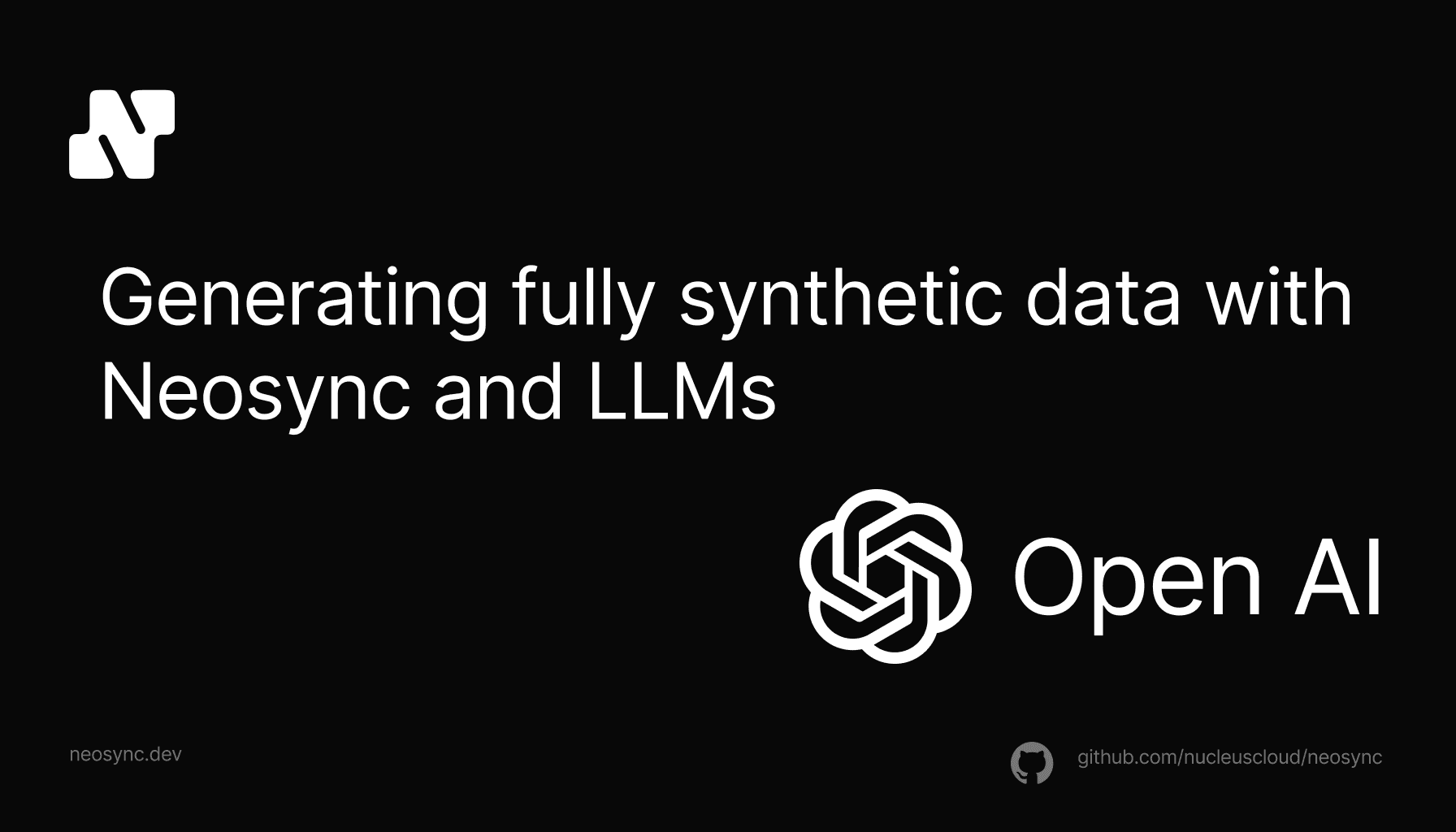
Generating Synthetic Data with LLMs
A guide to using AI to generate synthetic data for your database and application using any LLM that is available at an endpoint.
May 21st, 2024
Test data is one of the most important yet least talked about parts of software engineering. At a certain point in time, every developer has thought that their code was perfect and would function without bugs. Then they wrote some test data and realized that some bugs only appear when you start to put data through the system. Whether it's type mismatches, state management issues, scalability and performance bottlenecks or something else, testing your code with data is the only way to be certain that it actually works. So let's talk a look at what test data is, how developers use it and how to create it.
Test data is a set of data that is designed test the functionality, performance, and security of an application. The idea is to simulate, as closely as you can, real-world data that your application is expected to encounter when it's live. There are few different types of test data:
Depending on the feature and test case scenario, one of these types might make more sense than the other. Or, often it's a combination of different types of test data that you need to sufficiently test your application. We'll look at some examples below.
Test Data Management refers to the overall workflow of generating and managing test data. The goal is to ensure that test data is accurate, secure and effectively managed in order to ensure that developers are able to confidently test their applications. For example, say that a team of developers pulls data from a staging database to develop locally. Later, they submit a pull request for their feature only to see that their test are now failing. But it works locally, so what could be the cause? One possible issue is that the test database and the stage database are using two different datasets. In order to verify this, a test data management platform can help to sync data across environments so that the developer can narrow down the root cause and fix it. This versioning and syncing are core features in test data management among other things such as data anonymization, subsetting and validation.
High-quality test data is essential for several reasons:
Now that we have a pretty good understanding of what test data is and why it's important, lets take a look at how it's used. Test data is and can be used throughout the entire SDLC to ensure that your application is ready for production use. Here are some ways to use test data:
There are many different ways to create test data depending on what your want to test. As we mentioned above, different scenarios call for different types of test data. For example, static test data is fairly straight to create because it doesn't change often and can easily be reused. On the other hand, production-like is much more difficult to create because you need to think about the security and privacy concerns of the data leaving a secure environment. Here is how to approach creating test data:
Identify how the data will be used. This will help you decide the type of test data you need.
Once you've narrowed down the type of test data you need, the next step is to define what the data should "look" like. These are the characteristics of the data such as the format, size, distribution, validity, etc.
Now that have an idea of the shape of the data, it's time to generate it. If you only need a few rows of data the, then it might be sufficient to just write it by hand. If you need more data, then there specifically designed tools that you can use depending on your use-case. Here are some suggestions:
Neosync is built to create production-like test data that developers can use to test their applications. The best part is that it's all self-serve and developers have complete flexibility over the schema and how the data is generated. Neosync ships with 40+ Transformers out of the box or developers can create their own using custom javascript.
Test data can be created by anonymizing existing data or generating net-new data using Neosync's synthetic data generation.
Test data, though often overlooked, plays a crucial role in building secure and resilient applications. Whether you're training machine learning models or testing a SaaS application, test data can be the difference between a great user-experience and a not so great one.
You can get started with a free Neosync account by signing up here.
A guide to using AI to generate synthetic data for your database and application using any LLM that is available at an endpoint.
May 21st, 2024
What is the best way to protect sensitive data in LLMS - synthetic data and tokenization? We take an in-depth look at the two options.
April 23rd, 2024